Relevance
The document delineates a data quality framework for varied research domains, establishing criteria and standards for data quality. By uniting domain experts, infrastructures like BBMRI-ERIC, standardisation organisation and projects, it aims to streamline quality management and standard identification, showcasing the intricate and diverse landscape of data management practices. This work is significant for standardising data quality in the research community, fostering more reliable and interoperable research outputs.
Scope
The document from the EOSC-A Data Quality subgroup, underscores the paramount importance of data quality within EOSC for upholding the credibility, legitimacy, and actionability of resources. It aims at establishing certification and conformity mechanisms to ensure research infrastructures adhere to explicit rules, thereby assuring researchers about the professional management of their data and mitigating potential data sharing barriers. This effort is grounded in systematic literature review and community engagement, including surveys and case studies, to identify pivotal concepts and devise actionable recommendations.
Main highlights
The document “Towards a Data Quality Framework for EOSC” underscores the criticality of data quality, detailing standards and processes for ensuring data credibility, legitimacy, and actionability. It highlights the formation of agreement on compliance and conformity mechanisms to bolster researchers’ confidence as a trusted pillar in data management. Recommendations from systematic literature reviews and community consultations are formulated, emphasising the necessity of aligning data characteristics with requirements for diverse applications and stakeholder needs. The framework aims at facilitating community consensus on standards for the web of FAIR research data, enhancing dataset structure and documentation for usability, and advocating for direct involvement of data producers/providers in quality improvements. This effort seeks to raise awareness on data quality expressions across scientific domains, proposing a governance model that could significantly influence data management practices and policies within and beyond EOSC’s scope.
Key recommendations
The document offers comprehensive recommendations for enhancing data quality within the European Open Science Cloud (EOSC):
- Data quality assessment needs some minimalistic (fir for use) but fundamental “tests suits” to check data against;
- Data in EOSC need to be served with formalised and harmonised information for the user to understand how data quality is managed;
- Errors found by the curators or users need to be rectified by the data producer/provider;
- User engagement is necessary to understand the user requirements;
- Develop a proof-of-concept quality function or indicators performing basic quality assessments tailored to the EOSC needs
- Data quality is a concern for all stakeholders;
- Further refinement will be necessary, especially for AI training data or representational objects, and specific declaration of standard compliance;
- Will need to be identified. Such data quality declaration could be promoted as Unique Selling Point for EOSC and its wide covered scientific domains.
Co-chair contact
Support officer contact
Type of result
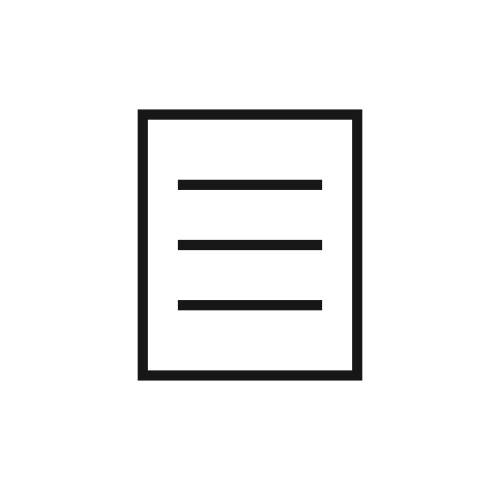